The last decade saw significant investments in cloud-based data consolidation across industries, including biopharma. Data “lakes” and “warehouses” became ubiquitous and markers of innovative thinking. The idea was that having centralized data would enable more efficient and intelligent business decision-making. However, the immediate value of this mass data consolidation was limited, in part because the gap between data consolidation and analytics was wider than most IT practitioners understood at the time.
So, was the data consolidation craze a bust? In the sense that it wasn’t the panacea many practitioners expected, yes. But all wasn’t lost. There’s no doubt the move to centralize data management was vital. However, instead of offering the complete answer for companies looking to improve business intelligence and find that elusive “single source of truth,” data consolidation was a steppingstone to a higher level of data analytics maturity.
The time to achieve that higher level of data analytics maturity is now. Today, companies are able to bring together advanced cloud data management capabilities with data science expertise and analytics techniques (think machine learning and artificial intelligence) to leverage consolidated data, generate market insights and extract business value from large data sets. Therefore, cloud-based data consolidation is a piece of the puzzle, but companies must complement it with enhancements in data science expertise and analytics capabilities. Then, they must bring these data management and analytics capabilities together and create an iterative feedback loop between data science and analytics on one side and data management and engineering on the other. With a unified, cloud-based data management and analytics infrastructure, companies can uncover the right insights, properly disseminate those insights across their organizations, and take action on those insights.
The drivers of cloud-based PaaS in biopharma
Platform-as-a-service cloud technologies (from Amazon Web Services to Microsoft Azure) offer several important benefits for biopharma companies. For example, they:
- Enable data consolidation and support efficient data management.
- Easily scale to rapidly process data through the use of parallel processing.
- Provide virtually unlimited and on-demand resource capacity.
- Offer unprecedented access to cutting-edge technologies and services.
There are two major factors driving the need for biopharma companies to put in place robust and sophisticated cloud data management infrastructures. First is the persistent growth of data in the industry. This is not a new story, but it remains an important one. Biopharma companies need to organize and integrate ever larger and more varied data sets to facilitate analytics and insight-gathering. Commercial leaders must enjoy seamless and timely access to data and insights in order to recognize opportunities quickly and agilely shift strategies in line with market changes.
Alongside the growth of data in the industry come shifts in insight-gathering capabilities and goals. Increasingly, companies are able to take a more predictive posture to their analytics. Instead of simply taking a retrospective view and figuring out, “what happened?” companies can seek to understand what they should be doing to gain an edge in the marketplace.
Advancements in data science capabilities and analytics methods enable this more sophisticated insight-gathering. However, an optimized cloud data management infrastructure is a crucial prerequisite for success.
The keys to cloud data management success
When working to implement a new cloud data management infrastructure or optimize an existing one, biopharma technology and commercial leaders should keep in mind a few keys to success:
- Understand the business objectives: Behind any cloud data management and analytics project are a set of business questions commercial leaders hope to answer and processes they need to support. These goals are foundational in informing the overall architecture as well as specific integration details. Therefore, they should be front and center throughout the project.
- Treat data as an asset and manage its lifecycle: A company must view its data as an asset to properly manage, protect and let evolve. The starting point here is to understand the full lifecycle of data; this includes the ingestion of data from various sources, storage of raw and processed data, data transformations and quality checks, data processing, movement of data between systems, and ultimately how data is safely removed or destroyed. Proper versioning and lineage allows scaling to multiple teams, trend analysis, quality control, and the troubleshooting of any data errors. Security, data resiliency (i.e., replication and backups), and access considerations also need to accompany each step of the data lifecycle. And business objectives should govern a company’s actions throughout the lifecycle.
- Adapt IT fundamentals for a new ecosystem: The IT fundamentals (data security, availability, access rules, segmentation, backups, logging, monitoring, etc.) that apply in an on-premise setting are to a large extent the same in a cloud setting, but the specifics differ and can even vary across cloud platforms. It’s important to remember that the cloud security model is a shared responsibility model. Cloud providers ensure security of their data centers, but companies still have to secure the data they store in the cloud by configuring their systems properly and maintaining appropriate controls. IT teams should revisit and rework established practices, as needed, for the cloud space while keeping in mind that the fundamentals cannot be compromised.
- Provide repeatability: It’s important to have mechanisms in place to efficiently create and maintain cloud infrastructure as systems scale and more components and services are utilized. A company also can’t afford to have gaps in cloud configuration or security protocols because of employee turnover. Utilizing automated methods and maintaining detailed playbooks help reduce operational effort and ensure all cloud data management practices are followed and remain easily accessible to anyone who joins the IT team.
Another key point commercial and IT leaders must keep in mind is that a cloud data management project is always more complex (and requires more decisions) than project leaders realize at the outset. Therefore, it’s important to build a cloud data management infrastructure deliberately and with conscious planning for ongoing maintenance, while also growing and maintaining the right set of skills and expertise along the way.
The analytics integration
To maximize ROI from data investments, a company must stand up a robust data analytics infrastructure that seamlessly integrates with its cloud data management infrastructure. This integration will enable commercial teams to uncover valuable insights, share those insights with the appropriate stakeholders, and then take action that improves commercial effectiveness.
As with cloud data management, everything comes back to business goals. So, when developing an analytics infrastructure, the biggest question a team needs to answer is, “What are we trying to get out of our data?” The answer to that question should reveal the overarching goals behind a data analytics effort. It can also help a company determine the type of data analytics technology it needs (there are plentiful options, from business intelligence tools like Tableau and PowerBI to machine-learning platforms like Amazon SageMaker to options that facilitate flexible coding like Databricks). Further, the answer should prompt follow-up questions, such as:
- Who are the consumers of our data and insights?
- What is the most effective way to deliver these insights, and how often do our stakeholders need to receive insights?
- Can we identify if the insights are being acted on?
- What talent do we need to add to the team (either through hiring or through consulting partnerships) to run our data analytics efforts?
It’s also important to include analytics as part of the data management lifecycle to properly protect that data and provide a potential feedback mechanism for refining insights. The bottom line is that a biopharma company needs to ensure its data ingestion and processing infrastructure fits together with its data analytics infrastructure to support executing on business goals.
Improve commercial effectiveness with the cloud
Every company is on its own cloud data management and analytics trajectory. A biopharma company that is launching its second or third product likely has a well-established cloud data management and analytics foundation. But a company preparing for its first launch may still be making preliminary technology decisions and working out its cloud processes.
No matter where a company lies along the cloud maturity spectrum, deploying cloud technology in a way that maximizes commercial ROI requires advanced planning, strategic investments in both people and technology, a laser focus on business goals, and a commitment to IT fundamentals.
As we move beyond what we’ll call the “data consolidation era” and into an era when biopharma companies will be able to more effectively leverage advanced analytics to support more agile commercial operations, it’s important that IT and commercial leaders understand the crucial role of cloud data management and analytics in improving commercial effectiveness. By putting in place and integrating robust cloud-based data management and analytics infrastructures, biopharma companies will put themselves in position to optimize insight-gathering and maximize commercial gains.
- Carl Onak
- Carl Onak is director of data engineering at Beghou Consulting.
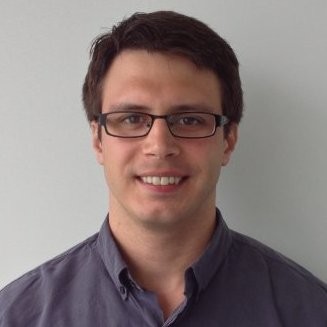
- Chris Weathers
- Chris Weathers is director of cloud services at Beghou Consulting.
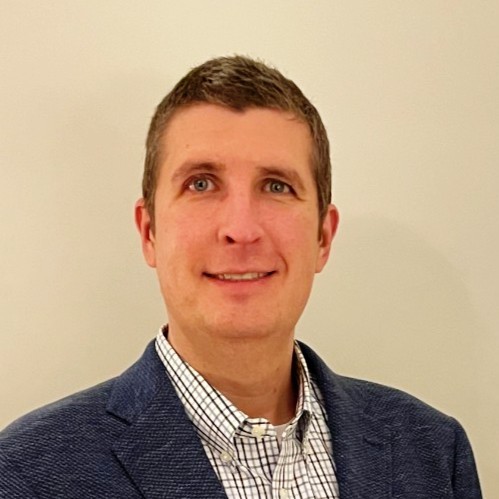