Earlier this season, the Tennessee Titans faced a fourth down and two in overtime at the Philadelphia Eagles’ 32-yard-line. If Titans coach Mike Vrabel followed conventional wisdom, he would have sent kicker Ryan Succop out to attempt a tying field goal. Instead, Vrabel went for the first down, a decision that paid off as the Titans converted and went on to score the winning touchdown. To the average fan, Vrabel’s decision seemed risky. After all, if the Titans failed to convert, the game would be over. However, Vrabel’s decision was, statistically speaking, the correct one.
There’s a good lesson for life sciences companies in this story: Be bold enough to trust the seemingly counterintuitive advice that data can provide. When a life sciences company sizes its sales force, it must assess many variables and make important assumptions that can significantly impact the number of territories it decides to cover. The only way to do this effectively is to deploy advanced analytical techniques that generate actionable predictions. Combining these data-driven predictions with judgment and experience is key to commercial success.
While many life sciences companies leverage machine learning techniques to determine their targeting and sales force deployment strategies, they too often leave these tools on the bench when it comes to sales force sizing. And the analytical methods they use for sizing tend to rely too heavily on human intuition. For example, a company may segment health care professionals by specialty area and account for a few variables in its regression analysis, such as a physician’s total prescriptions or the number of sales calls the company made to that physician over the past six months. This approach can provide some answers. But it’s tough for a company to get all the answers it needs when there are myriad other variables it should have considered. As a result, companies may be leaving opportunities on the field at launch.
The simple truth is that human insight – even from the most seasoned analytical professional – is limited. But combining human insight with machine learning allows a company to incorporate significantly more variables in its models, eliminate bias, and enhance accuracy and predictive power.
- STEVE TROKENHEIM
- Steve Trokenheim is a partner at Beghou Consulting.
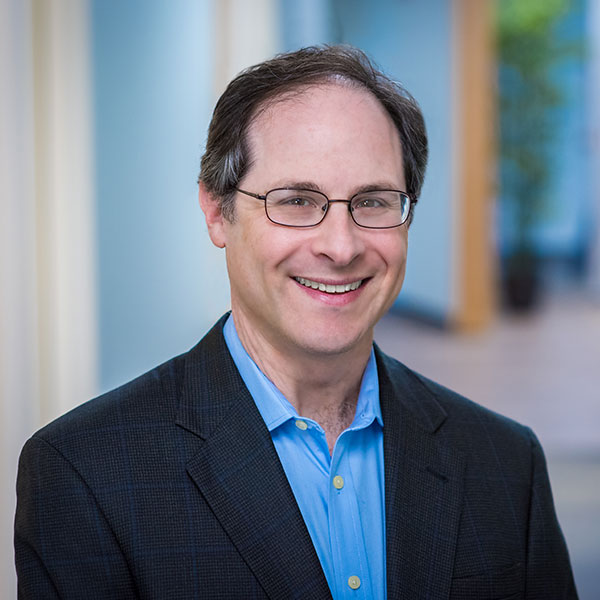
- ROB LIEN
- Rob Lien is an associate partner at Beghou Consulting.
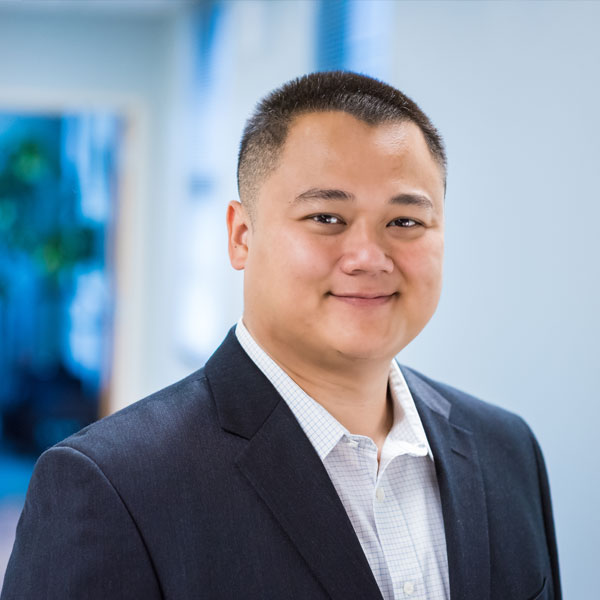